Finding an open parking spot can be a huge headache for drivers in metropolitan areas. Yet, in a seemingly contrary statistic, US cities have far more parking spots than are needed. Take Seattle, for instance – according to the latest research, the city has over 1.6 million parking spots. That equates to about 5 parking spots for each household in the city!
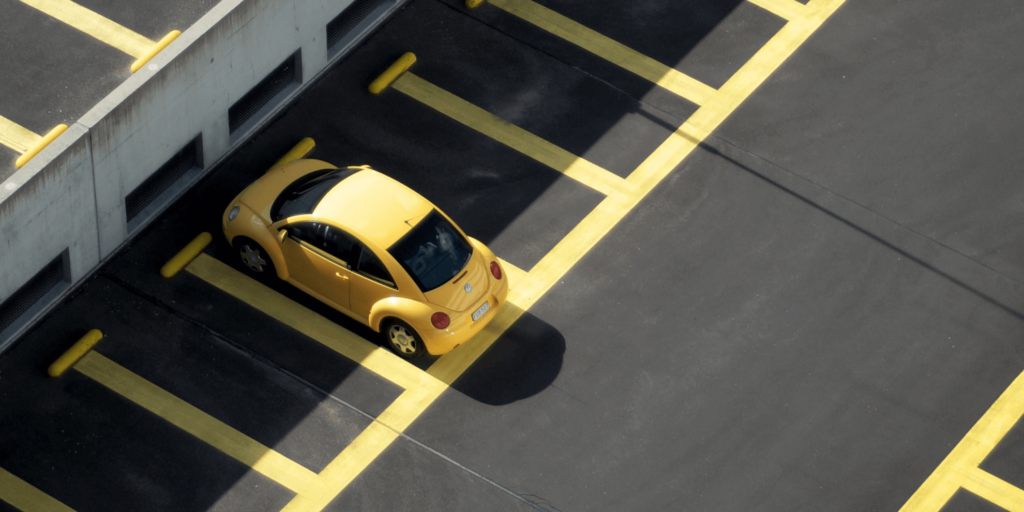
And that’s just a mild example. Des Moines has an astonishing 19.4 available parking spots per household.
With such an overabundance of designated parking spaces, why is it so hard to find a place to park? The answer is simple: unintentional ignorance. City dwellers all have their favorite parking spots – ones that have clear signs and are easy to access. Less popular parking spots might be a little more difficult to notice, but they are often full of vacant spots.
In a study from Bloomberg City Lab, it was found that even though 27 studied districts had oversupplied parking, there was a public perception of scarcity. But in actuality, supply outweighed demand by an average of 65%. That’s a lot of empty spots!
So, how do we go about making vacant parking spots more accessible to the average driver – and in a way that alleviates the burden on city planning? One way is to predict available parking spaces, which allows drivers to see which spots will be vacant upon arrival and use that information to plan their route. This is made possible through Machine learning (ML) technologies, which assess how the parking situation will change in an hour and act accordingly.
Smart cities – urban areas that use data-driven insights to efficiently manage public services – stand to heavily benefit from parking space prediction. If drivers have access to a solution that predicts upcoming vacancies, the city’s traffic congestion and pollution will decrease – and, what’s more, the Smart City planners can use the rich data to drive future infrastructure decisions.
This is where Intetics’ data science approach comes in. We’d like to present you with three cases, in which Intetics created ML-powered parking solutions.
Case 1: Parking Spot Detection via Parking Sensors
In this case, the customer wanted to know how many parking spots (and which ones) would be free/occupied 14 days in advance. Intetics analyzed a couple of months’ worth of data from the client’s parking sensors, using it to build a basic prediction model. In order to improve the model’s accuracy, Intetics added external factors like the weather, a calendar of holidays, parking location, etc.
As an output, the client received a convenient web application, in which users can track predicted parking occupancy – for each slot and in general – for the day.
Case 2: Parking Spot Detection via IP Camera
In a similar case, a client wanted to know which parking spots would be free/occupied 14 days in advance; but in this case, the integrated sensor solution was too expensive and time-consuming. So, instead, Intetics worked with the client’s existing hardware, a security IP camera.
The customer received a module with real-time occupancy tracking, which gathered enough data for model training. Then, Intetics used the results to develop a pipeline for occupancy prediction.
Case 3: Model Development for Automatic Recognition of Parking Signs
In this case, a client required an ML pipeline to detect available locations for on-street parking, based on the parking sign’s location, and recognize its restrictions and time limits. The solution needed to have at least 90% accuracy, and it should be able to recognize text on unstandardized parking signs.
Intetics’ main objective was to create an ML model and wrap it into a stand-alone web service for automating the collection and entry of parking data. In turn, that service needed to be integrated within a customer analytics platform.
When building the solution, Intetics used semi-automatic data labeling. As it is much faster than manual data labeling, Intetics was able to reduce time spent by 70%. Then, the data was used to train a Machine Learning model – which led to 90% recognition accuracy.
All of the ML and data processing algorithms were implemented into a single web app, providing the client with automated on-street parking locations detection and a significantly reduced workload.
The Science Behind the Solutions
Predictive modeling is a statistical technique used to predict future behavior. Data-mining solutions analyze current and historical data, thus generating a model that can predict possible outcomes with the high level of certainty. The components necessary for an advanced predictive parking system include: a sensor, gateway hardware, a server/cloud backbone, and a mobile application
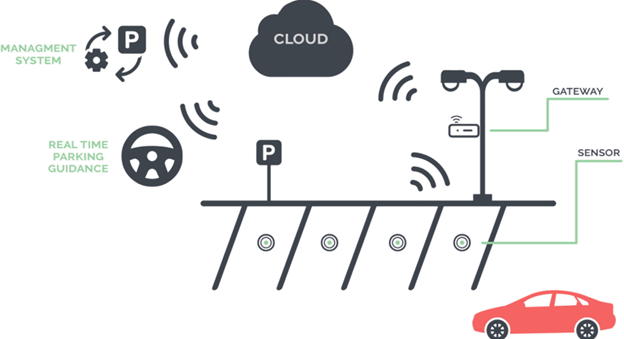
In this manner, data is collected (using sensors or IP camera), a statistical model is formed (parking patterns), predictions are made (free/occupied spots 14 days in advance), and the model is revised or validated as additional data comes in (holidays, weather, etc.).
The Scope of Predictive Parking
Urban mobility is an essential component of smart cities. Due to the rapidly increasing number of vehicles, metropolitan traffic congestion is growing worse – in fact, drivers that are searching for parking spaces contribute to traffic congestion by up to 30%. The spots are available, but finding them is the hard part.
By cutting down on the average time spent seeking a parking space, drivers will be able to reduce direct and indirect costs of looking for a spot. In the US, motorists waste an average of $345 in fuel and lost time per year, all from searching for a place to park. In the UK, the search for parking rises to 733 GBP ($1,013.30). And in Germany, motorists waste 896 EUR ($1,056.16) per year. This could all be dramatically reduced with the use of a predictive parking app.
Beyond that, there is also the environmental impact to consider. Spending more time looking for parking increases the emissions of cars. According to a study from the University of California, within a 15-block district in LA, drivers spend a combined 950,000 miles per year looking for parking spots. That is equivalent to 730 tons of carbon dioxide and 47,000 gallons of wasted gasoline.
So, for the good of the planet – and our wallets – it is necessary to predict which spots are open and where the lots are located. While clients might develop apps for individual parking garages or lot systems, city planning committees could very well decide to commission an app to reduce city-wide parking system complexity. And it makes sense; cities are becoming smarter in many areas: street lights, education, healthcare, homes, and energy are all connected to the IoT. Why not parking? As predictive parking apps become more commonplace, hopefully, circling the block looking for a spot will become a thing of the past.